Word Positions on the Folios – Conjectures
Conjecture #1: The Text is Song/Poetry Transcriptions
Rhymes in songs and poetry feature words at the ends of lines that rhyme with other line end words. So a plausible explanation of why we see certain words mostly appear at the ends of lines is because they are words that easily rhyme with others.
Conjecture #2: The Words in the Text are Codes
We know that the VMS word lengths follow a binomial distribution, which suggests they are actually numbers. The fact that many words have the property that if you remove the first glyph the resulting word is also valid (i.e. observed in the text) strongly supports this. E.g. the word “12345” gives “2345”, which is a valid number.
Conjecture #3: The Text is a Transcription of Old Occitan
Old Occitan, which was on its way out at around the dating of the manuscript, is a nice candidate source language for various reasons (which I will leave for later). The Conjecture is that the VMS text words are numbers (Conjecture #2), which when looked up in a (long-lost) dictionary, equate to Occitan words. Since many of the existing manuscripts in Old Occitan are records of songs sung by the troubadours, this fits with Conjecture #1.
Word Positions on the Folios – Part Deux
In the previous post, we looked at Voynich words that have a marked affinity for the first position in a line of text, with a restriction to words on the Currier B folios. Those words are:

In this post, we’ll look at the words that have an affinity for the ends of the text lines, and words that are disinclined to appear line initial or terminal.
For this, we introduce the metric that is the fractional position of the word in the text line. For the word at the start of the line, this metric is 0.0, and for the word at the end of the line it is 1.0, and for a word half way along the line it is 0.5. We can plot this metric for each word.
Firstly, let’s look at the word “daiin” with the metric:
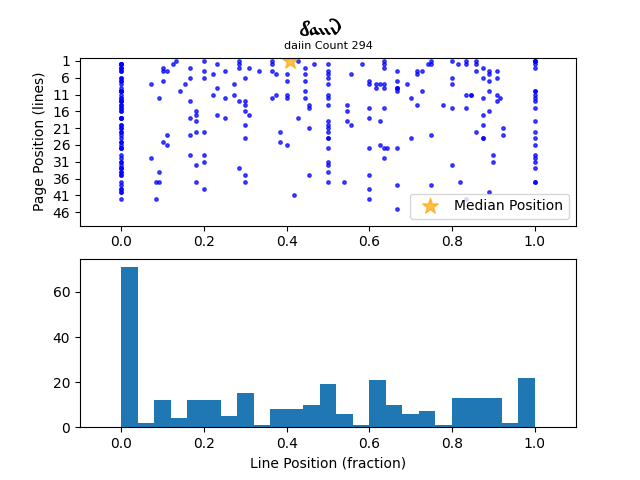
The upper half of the Figure shows the page positions of all instances of “daiin”, with the fractional line positions on the x axis. The lower half shows a histogram of the fractional line positions. We can see what we observed in the first post, that “daiin” has a strong affinity for the first line position (fraction 0.0). But we also see that it sometimes appears as the last position in lines of text (fraction 1.0).
Looking at all the words, we can categorize them into six categories as follows:
- Words often in First Position – dai, daiin, dair, sai, saiin, sar, sol
- Words often in Last Position – am, dy, oky, oly, qoky
- Words never in First Position – ai, aiin, air, al, am, chcthy, chdy, checkhy, cheedy, cheky, cheody, chody, chy, dy, kai, kedy, keedy, okal, okedy, oky, oly, opchedy, otai, otal, otar, oty, raiin, shckhy
- Words never in Last Position – ai, cheo, dai, kai, kaiin, okai, otai, qokai, qokain, qotai, sai, shckhy, sheedy
- Words never in First or Last Position – ai, kai, otai, shckhy
- The rest – ar, chckhy, chedy, cheey, cheol, chey, chol, dal, dar, dol, kar, lchedy, lchey, lkaiin, okaiin, okar, okeedy, okeey, ol, or, otaiin, otedy, oteedy, oteey, qokaiin, qokal, qokar, qokchdy, qokedy, qokeedy, qokeey, qokey, qokol, qol, qotaiin, qotal, qotar, qotedy, qoteedy, qoty, shdy, shedy, sheey, sheol, shey, shol, tedy
Words often in Last Position
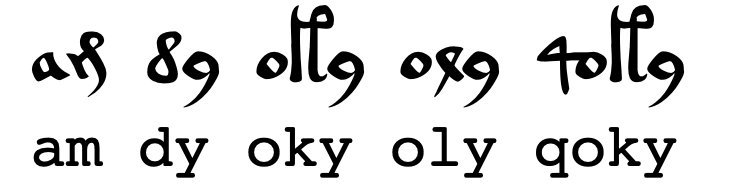
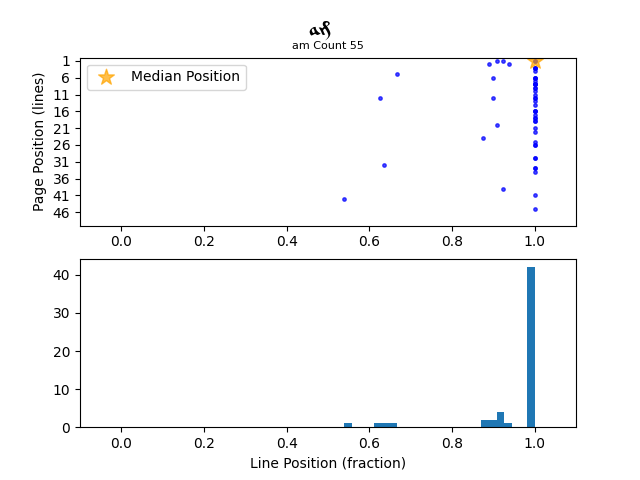
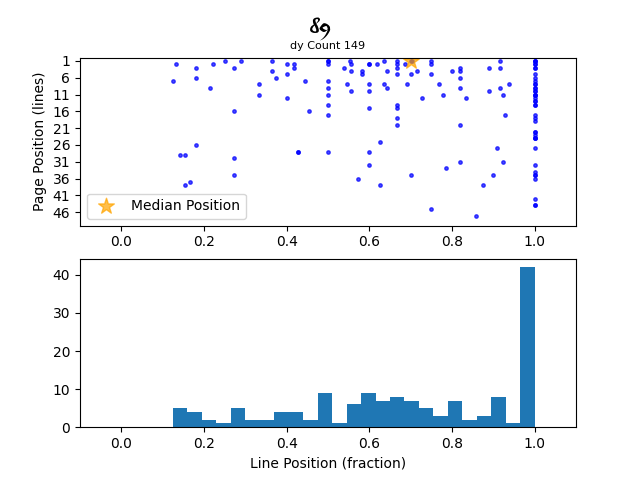


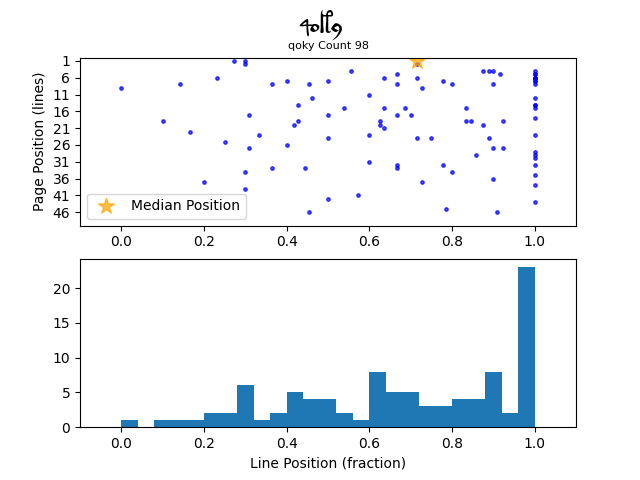
Words Never in First or Last Position

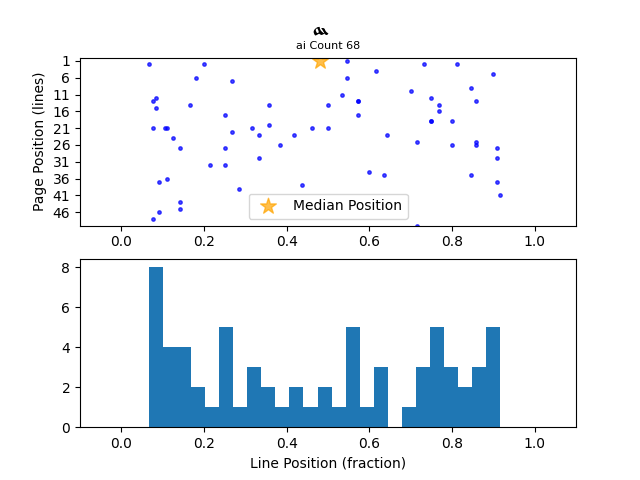
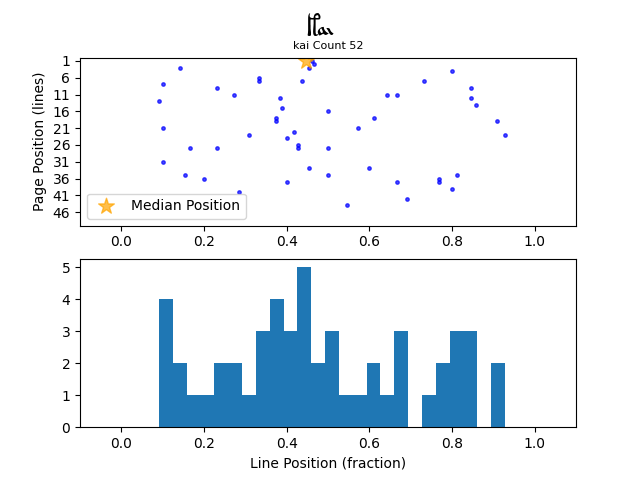
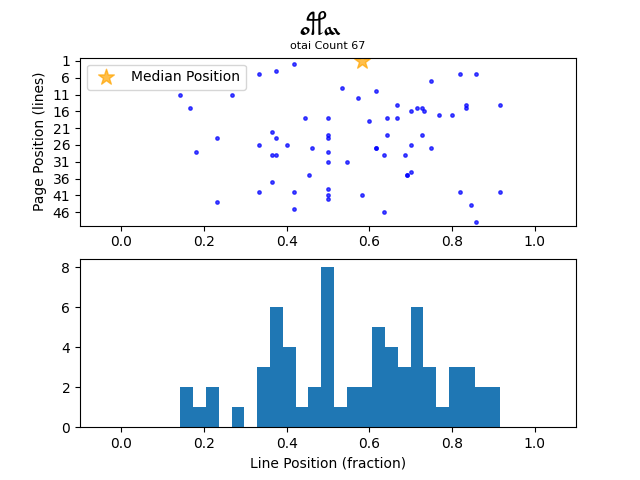
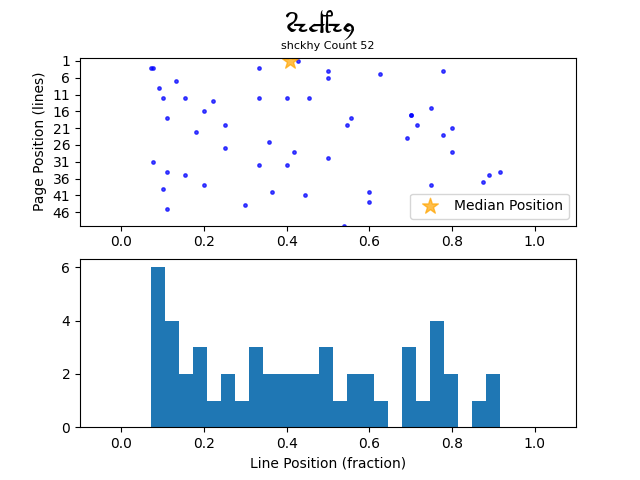
In the next post, we’ll look at possible explanations of these word positional data.
Word Positions on the Folios
One of the puzzling aspects of the text in the Voynich Manuscript (VMS) is the unusual positioning of some words on the folios. In particular, some words appear more often at the beginnings of lines, and some more often at the ends. For example, “daiin” shows a particular fondness for the first position in the line:
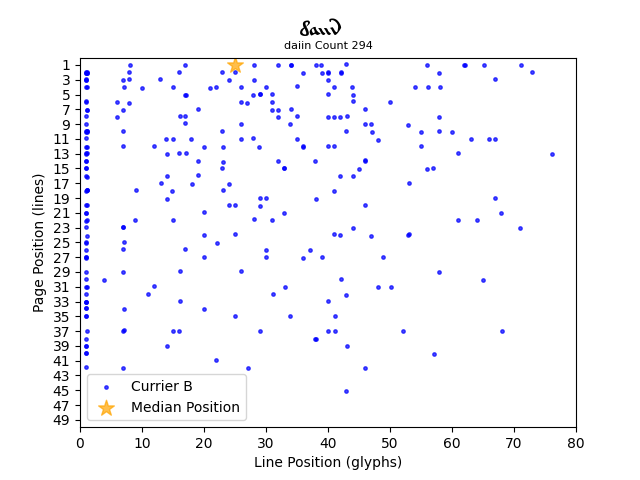
Whereas “aiin” shows a strong aversion for the first position:
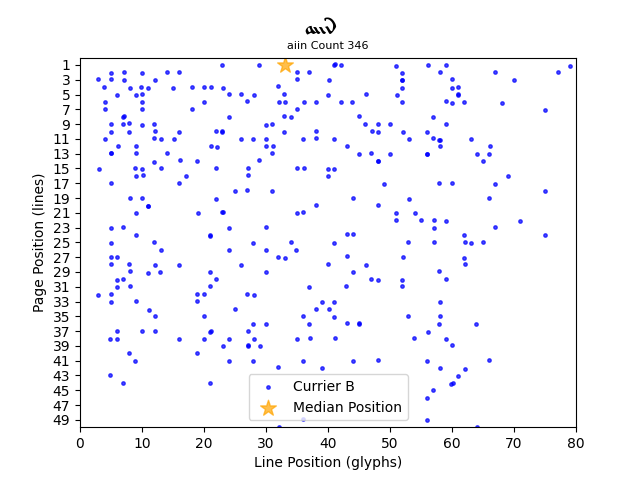
In contrast to “daiin” and “aiin”, the word “chedy” shows no preference for any particular position on the folio lines:
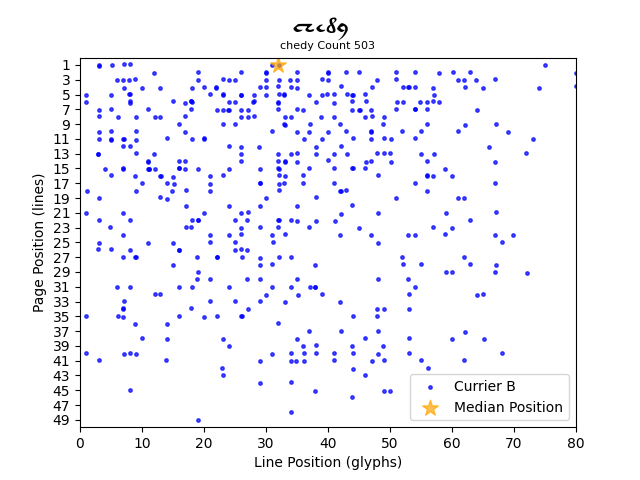
(There are some words that appear more often near the top of the folio, and some more often near the bottom, although this may simply be due to a topic change within the folio and a consequent change of vocabulary, so I don’t consider them further.)
These cases, the words that have an affinity for either the start of a line or the end of it, are curious and warrant some investigation.
For this study, I have restricted the data to only use folios in Currier B. For all words that appear on Currier B folios, I extract the glyph position of the word in the line:
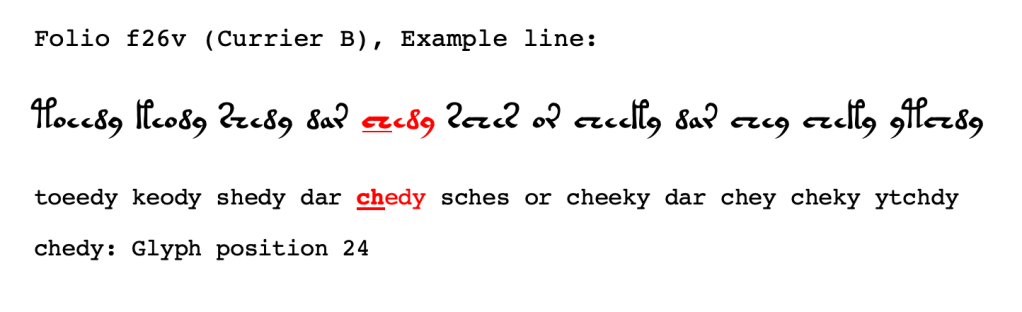
In the example above, the line of text contains the word “chedy”, shown in red, at glyph position 24. The data are further restricted so that only lines of text are considered that have at least three words, so as to avoid labels and other short text lines. Finally, only words of length at least three glyphs, and that appear at least 40 times over all folios are considered. For every word in the resulting dataset, and for every time it appears on a folio, the glyph and line position of the appearance are stored for analysis.
With the data, we can look at some metrics. A simple metric is the number of times a word appears as the first word in a line, Nf, as a percentage of the number of times it appears, N. Let’s call this metric PI = (100*Nf/N). If the word only ever appears as the first word, PI will be 100%, and if it never appears first, PI will be 0%.
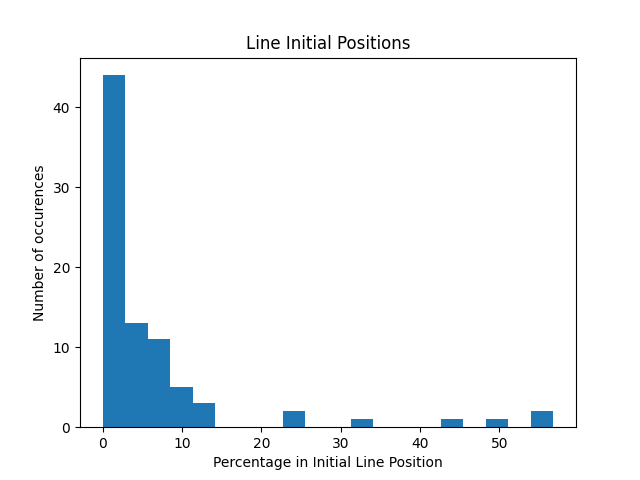
The histogram above shows the distribution for the metric PI. There are seven words with the property PI > 20% i.e. each of these words, when they appear, do so at least 1/5 of the time as the first word on folio line. The words are: dai daiin dair sai saiin sar sol

Here are the detailed folio distributions for each of these:
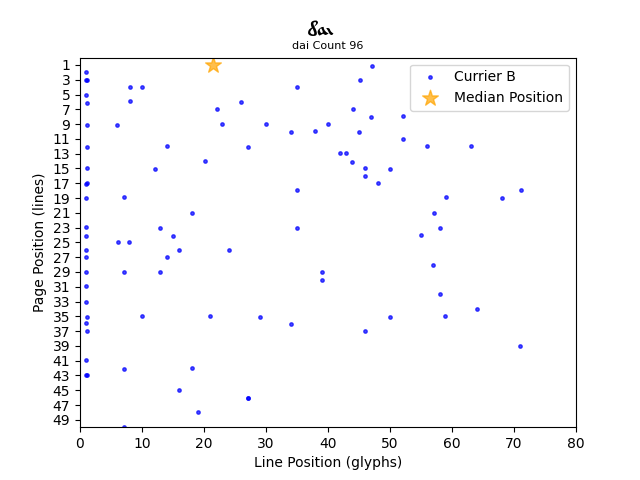
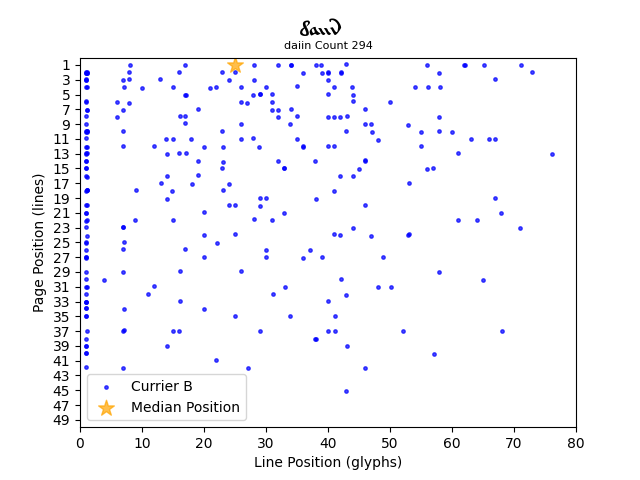

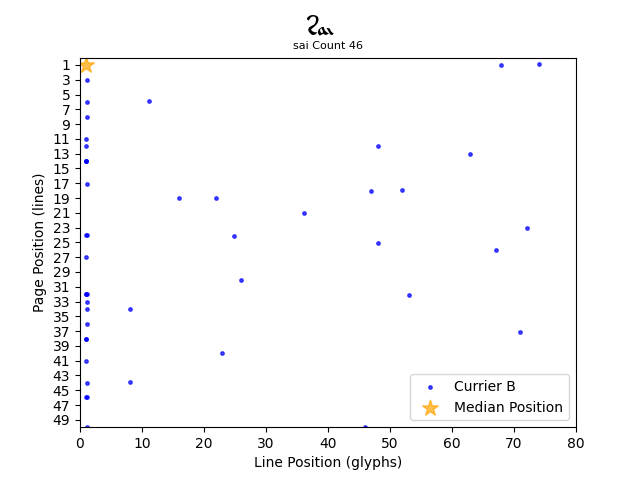
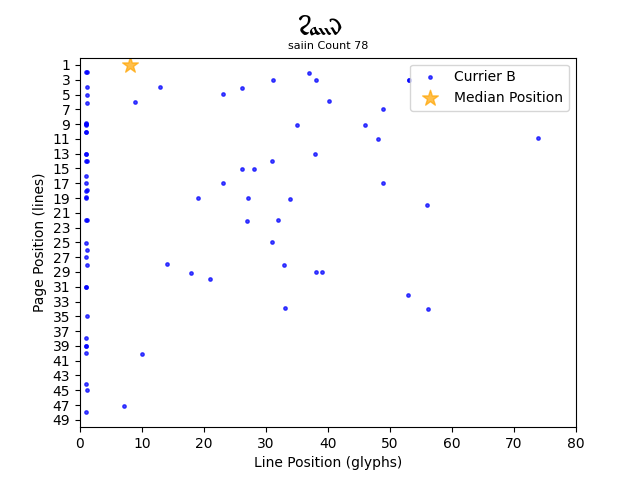

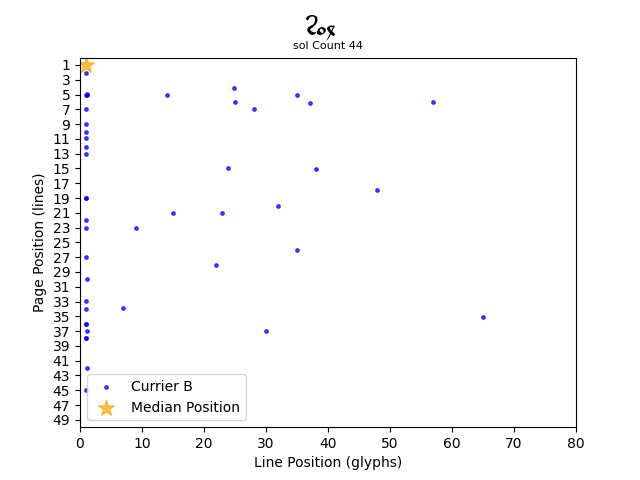
In the next post, we’ll look at VMS words that have an aversion to being first on a line, and then see if we can deduce any patterns between the two groups. What governs where a word is “allowed” to appear on a line – is it related to its component parts, i.e. does the word’s prefix/midfix/suffix dictate where it should appear on a line?
The Wheels hit a bump
To recap, the hypothesis is that the VMS text was written by use of a number of cipher wheels, each wheel containing a number of glyphs from which none or one was used. In addition, it was theorized that one of the wheels contained just the Gallows glyphs. The attractiveness of this hypothesis can be summarized as follows:
- The length of words created using a set of wheels in this way should be binomial distributed. This is the case, and was first observed by Stolfi.
- The number of words containing a gallows glyph should be about 50% (since the gallows wheel is chosen 50% of the time). This is approximately true (the VMS has about 60%).
- The number of words in the VMS that begin with a gallows glyph is about 13%, and this closely matches the number obtained when the wheel containing the gallows glyphs is the third wheel.
- The average length of a word that starts with a gallows glyph, should be shorter than the average length of all words that contain a gallows glyph (since the first two wheels were not used). This is also true, by one glyph on average.
- If the gallows glyphs only appear on one wheel, then gallows glyphs should never appear next to one another in a word. This is approximately true: there is one case in the VMS where two gallows glyphs appear together;
This may in fact be two words: EVA ot and EVA kchedy. (Aside: the challenge of deciding where one VMS word ends and the next begins is well known – what is a space, how big is it, and did the transcribers get it right?!)
So far, so good. But from the wheels hypothesis we can make another prediction: for words containing a gallows glyph, there should be at most two glyphs preceding the gallows, if the gallows are all on the third wheel. This is not the case: there are many words that have more than two glyphs preceding the gallows, even if you count some glyph combinations such as EVA qo and EVA ch, sh as one glyph.
Another prediction we can make is for the number of words that end with a gallows glyph. This number can be calculated from the wheel number and layout, and it turns out to be much smaller than what is observed in the VMS. Specifically, in the VMS, there are 85 words that end in a gallows glyph (about 1% of all words), but only about 0.1% are predicted.
Grove Word Lengths
In the previous post, we looked at how the Grove words (words with an initial gallows glyph) are distributed in the VMS, and how their frequency is explained by the use of cipher wheels to generate VMS words.
Marco commented on that post with the astute observation that if this generation scheme is valid then gallows initial words should be shorter than other words, on average, as only wheels 3 onwards are used to create them.
Here are the data: these show the lengths of Grove words compared with the lengths of other words that contain at least one gallows glyph:
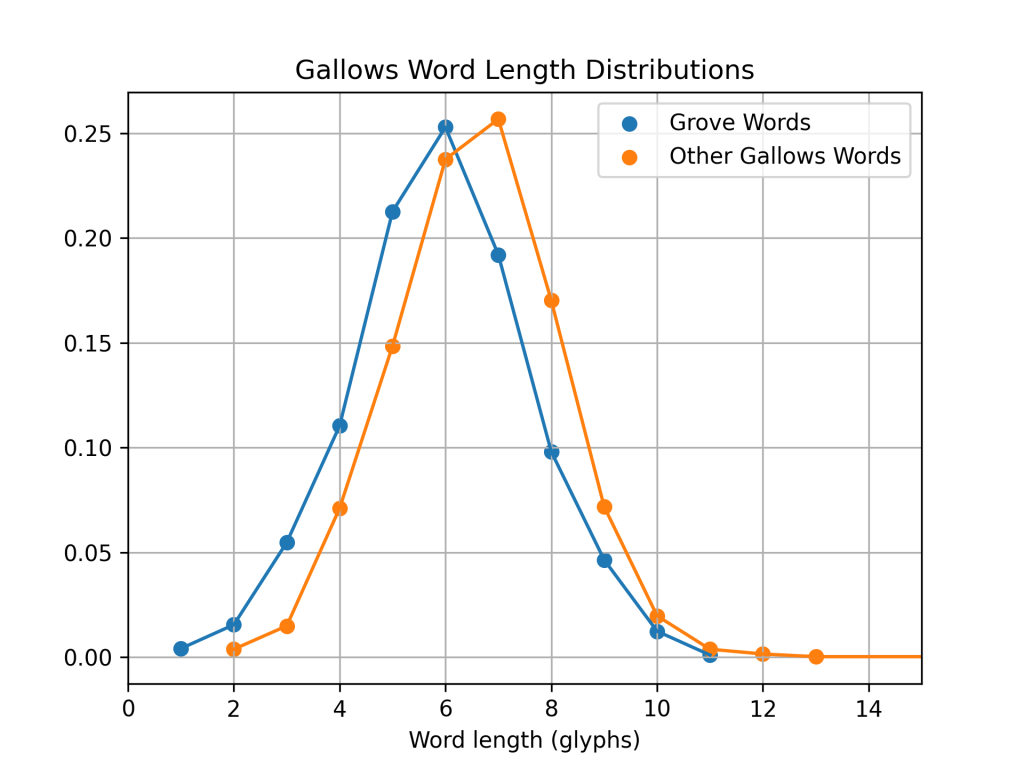
This confirms that, yes, Grove words are on average shorter than other gallows words (by about 1 glyph) – perhaps more evidence for the validity of this scheme?
For interest (as requested by Rene), here are the distributions for EVA l and EVA r:
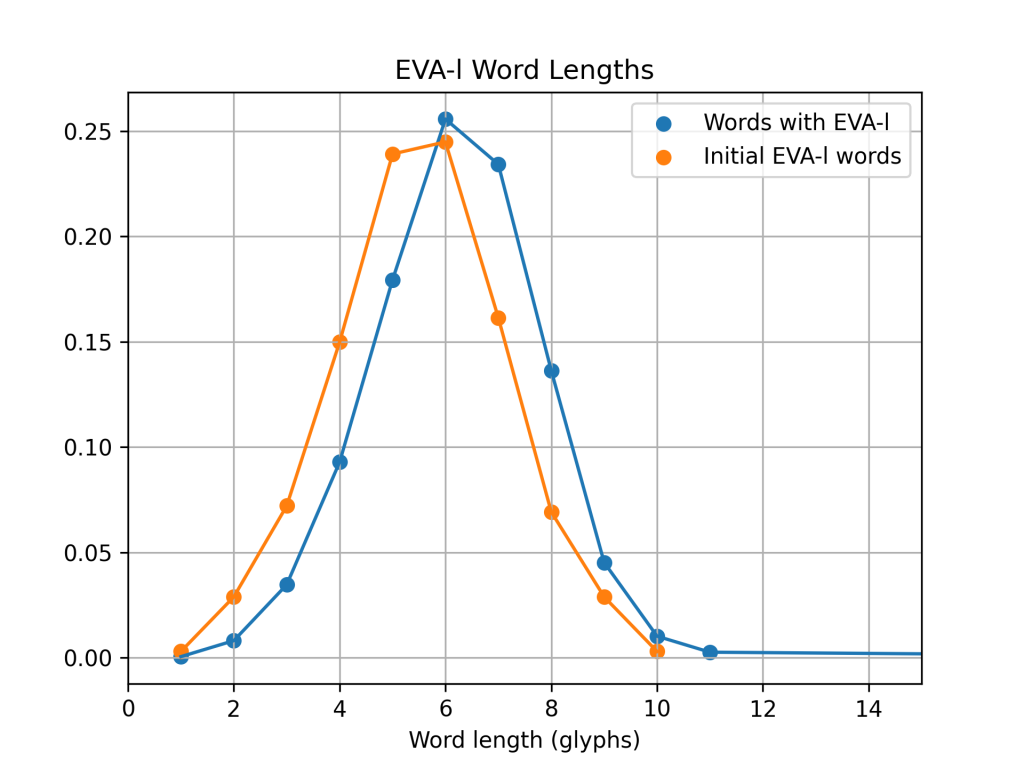

Fun with Grove Words and Cipher Wheels
What is a Grove word? The answer is a little fuzzy, but simplistically a Grove word is a VMS word that begins with one of the gallows glyphs. These words are often page or paragraph initial. Emma May Smith has a good explanation in her recent blog entry.
Mr. Grove observed the peculiar feature that some words beginning with a gallows glyph are also valid words if you remove the gallows glyph. For example, the word EVA kodaiin starts with gallows k, and odaiin is also a valid word.
It turns out that if you look at all words in the VMS, 46% of them have this property: remove the first glyph and you are left with a valid VMS word. Compare this with English, where only around 8% of words produce valid words if you remove the first letter. Making up the 46% we have 38% from non-Grove words (i.e. non-gallows initial), and 8% from Grove words.
To round out the statistics, about 13% of all VMS words have an initial gallows glyph.
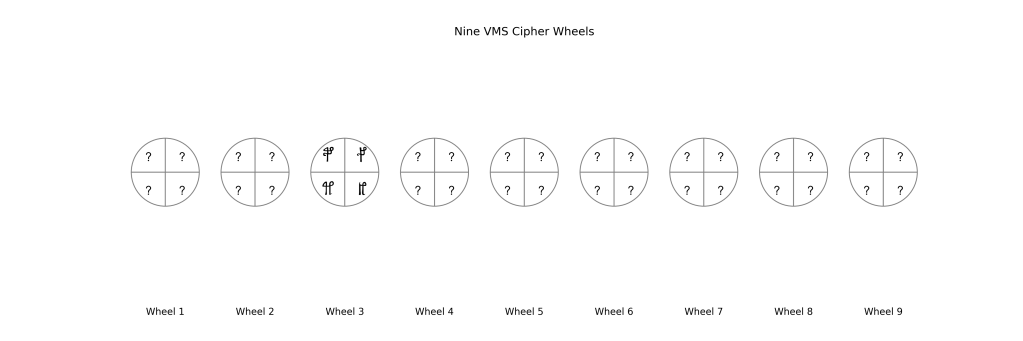
Consider the nine wheels above, where one of the wheels contains gallows glyphs, and the other wheels contain other glyphs. These wheels can be selected in 29 -1 i.e. 511 different ways, to make words of length between 1 and 9.
The probability of selecting wheel 3 as the first wheel for the word is about 12.5%. In other words, with these 9 wheels, 12.5% of the time we’d create a gallows-initial “Grove” word – very close to what we observe in the VMS (13%). In fact, this figure of 12.5% is independent of the number of cipher wheels: as long as there are at least three wheels and they are used left to right, and the gallows glyphs fully occupy the third wheel, then 12.5% of the generated words will be Grove types.
As a corollary, it’s clear that for Grove types generated with the wheels, removing the first glyph will produce a valid word, as it is equivalent to generating a word starting at wheel 4 or later.
So what of the 54% of VMS words that are non-Grove, i.e. removing the initial glyph does not produce a valid VMS word? This can be explained if the number of different words used and written in the VMS is simply less than the total number of possible words that the author’s wheels can produce. What is the expected vocabulary size if we know there are 7,552 words written in the VMS (Takeshi), and we are missing 54% of them? It is simply 1.54 x 7,552 = 11,630 words, or thereabouts.
(Aside: the wheels above could just as easily be represented and used as a table with nine columns.)
In summary, “Grove” words (gallows initial) are ~13% of all words in the Voynich manuscript, and this fraction is what you’d expect if the text was produced using cipher wheels.
Word Length Distributions
In the previous blog post, we looked at the distribution of word lengths in the EVA transcription, and compared it with the binomial distribution for 9, as per the work of Stolfi. They matched well enough, as I had denoted EVA ch, sh, ain, aiin and qo as single glyphs, in a similar fashion as Stolfi:
For this page, we will define symbol as Currier did; i.e. EVA ch ans sh will be counted as single symbols, and so are EVA cth, ckh, etc..
https://www.ic.unicamp.br/~stolfi/voynich/00-12-21-word-length-distr/
i.e. he reduced some of the EVA glyph sequences to single symbols.
Without making these reductions, so leaving the EVA transcription unchanged, the distributions of course tend to higher values. As a check of my sanity, Marco Ponzi was kind enough to send me a list of VMS words he’d extracted from the ZL transcription, so that I could compare it with the words I extracted from the Takaheshi EVA. In the following plot I show the three word length distributions: EVA, ZL and the reduced EVA with ch, sh, ain, aiin and qo as single glyphs.
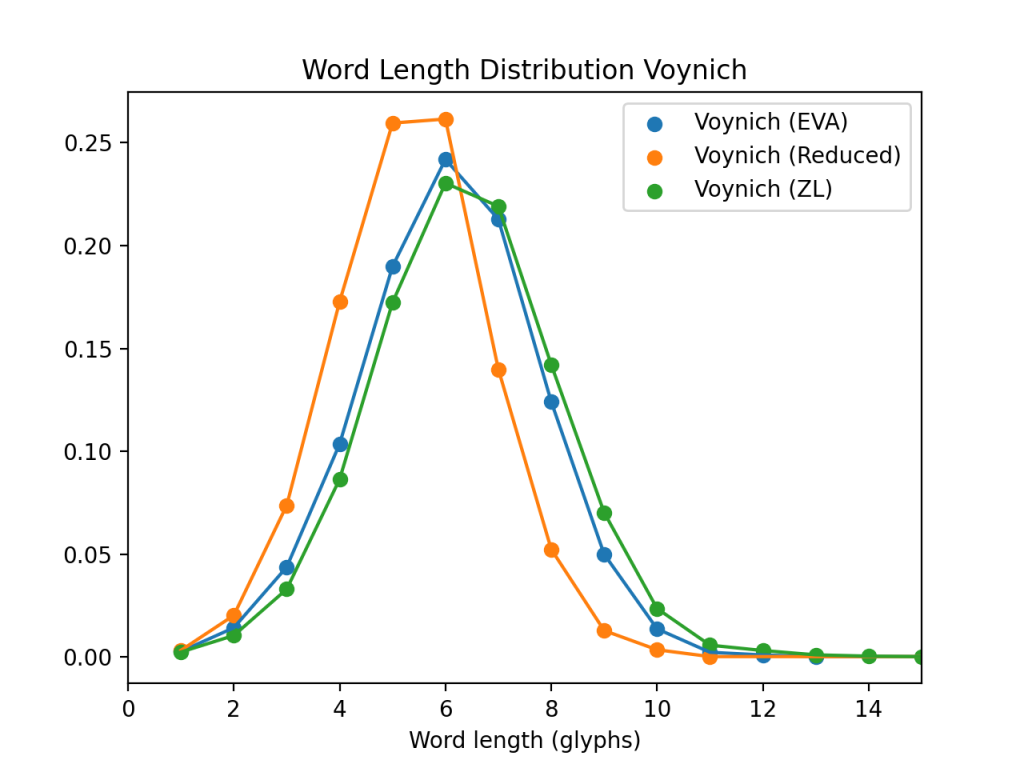
Reassuringly, the EVA and ZL (green and blue curves) match quite well, as they should, and the Reduced matches Stolfi’s result. (Curiously, the ZL transcription has a total of 8078 different words, compared with 7552 for Takaheshi EVA – which warrants further investigation.)
The EVA distribution now matches a binomial of (n=12,p=0.5), i.e. using 12 cipher wheels with a probability of 50% for a glyph being used from each wheel.
Nine Cipher Wheels
UPDATE (12 Aug 2021): the plots and results discussed in this post used a version of EVA that replaces some common glyph sequences by a single glyph, namely ch, sh, ain, aiin, and qo. Clearly, this tends to reduce the average word length. A later post will discuss the distributions obtained with words without this simplification.
The lengths of VMS words follow the binomial distribution for 9, as observed by Stolfi, and as discussed in Rene’s recent paper. This binomial distribution can be obtained from a set of 9 cipher wheels, where each wheel has a 50% chance of contributing one of its glyphs to the word being assembled, and the lengths of the resulting words plotted:

In the above plot, the orange line shows the distribution of word lengths from EVA, and the blue line shows the distribution of word lengths obtained by using the following set of 9 cipher wheels to generate a large number of random words:
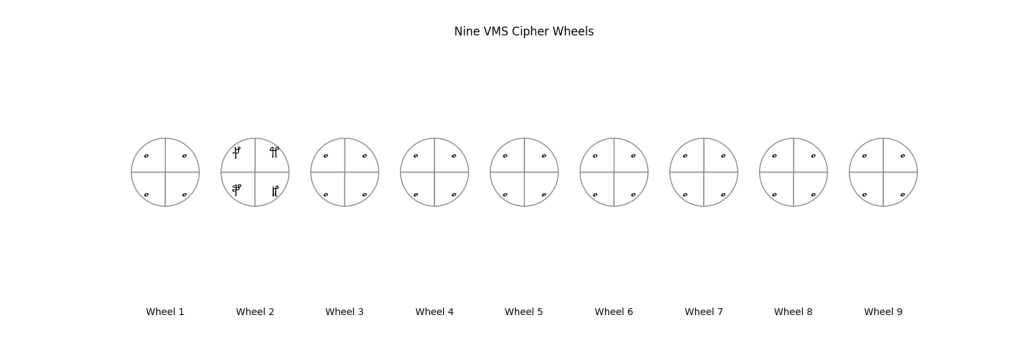
With the cipher wheels shown, about 50% of the generated words will contain a gallows glyph, and this is, perhaps not coincidentally, the case in the VMS text, too.
Using the same technique as applied in my earlier blog post, where I looked at the counts between gallows glyphs in the VMS text, we can look at the same distributions for words generated with the above wheels, assembled into lines of text, and ignoring spaces between words. The results are very similar, and shown below.
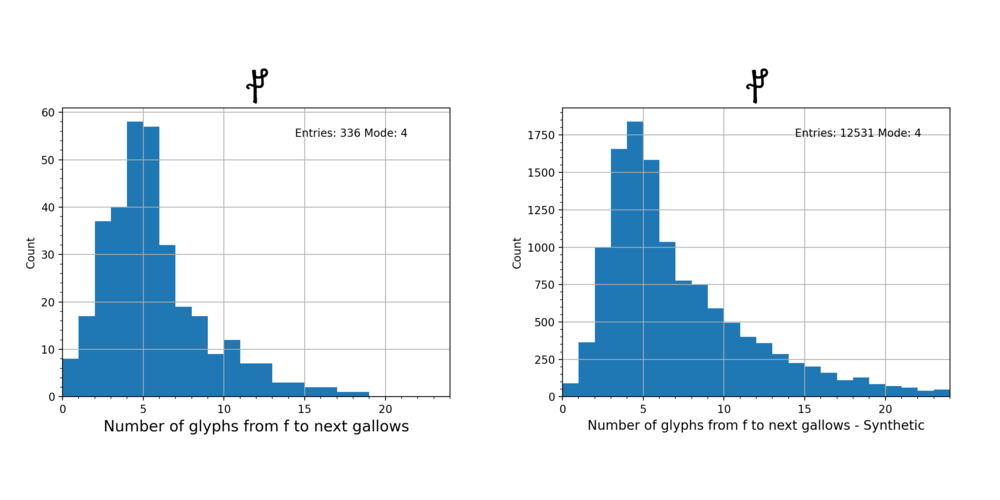
Here are the others:

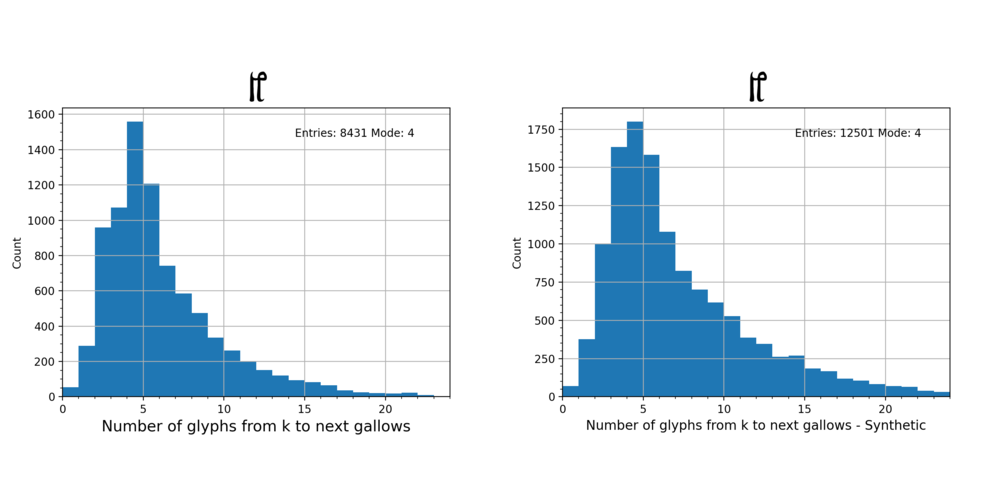
Cipher Wheels – Genetic Algorithm – Some Results
The latest run of the GA produced the following prediction after 5000 Epochs: 12 cipher wheels, covering 96% of all VMS words, as shown below.
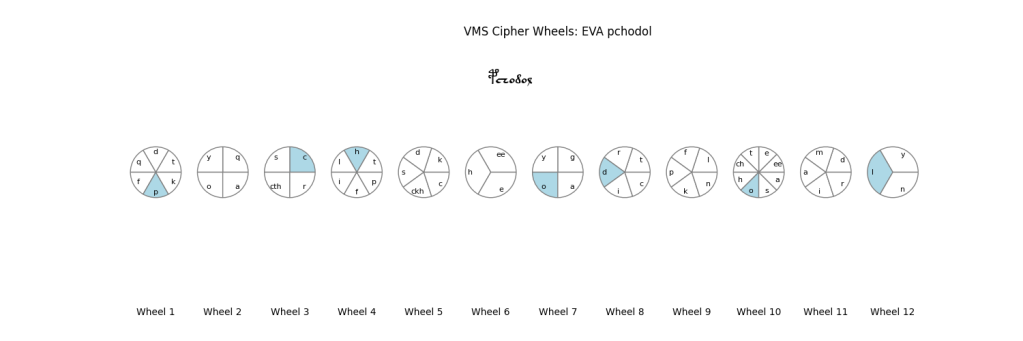
The GA was free to use up to 13 wheels, and as few as 3: these 12 are the best fit it found. The blue segments shown are those that can be used to create the example word “pchodol”, i.e. “p” from Wheel 1, “c” from Wheel 3, “h” from 4, “o” from 7, “d” from 8, “o” from 10, and finally “l” from 12.
There is some redundancy: another way of making “pchodol” is to take “p”(1), “c”(5), “h”(6), “o”(7), “d”(8), “o”(10), “l”(12).
Observations on the Wheel Configuration
The un-benched Gallows glyphs “p”, “f”, “t”, “k” all appear in the first Wheel. They also appear in Wheels 4 and 5, and Wheels 8, 9 and 10. It’s curious that the GA has divided them up in the later Wheels. Their appearance in the first Wheel covers the Grove words. Their appearance later allows for the Gallows word with preceding glyphs.
The GA has only found it necessary to include two of the benched Gallows: “cth” (3) and “ckh” (5). The other benched Gallows “cfh” and “cph” can of course be formed from “c”, “h”, “p” and “f” in the wheels. Why did the GA find it expedient to include “cfh” and “cph” as unique glyphs?
The GA was allowed to use the glyphs “in” and “iin” as if they were single glyphs, but it has not seen the need, perhaps suggesting that they are not single glyphs after all.
The existence of “ee” and “e” in Wheels 6 and 10 is probably a result of the scoring system employed, that tries to only take at most one glyph from each wheel, otherwise “ee” could be made simply by taking “e” twice – but that is penalised.
A Comment on Repeating Words
f75r in the VMS contains the infamous word sequence “qokedy qokedy qokedy”. The Wheels allow qokedy to be created in three different ways (shown below). This is thus a possible explanation of how that sequence occurs – when ciphering three different plaintext words using the Wheels, which Wheels and which positions to use will be different, but the end result is the same VMS word repeated.
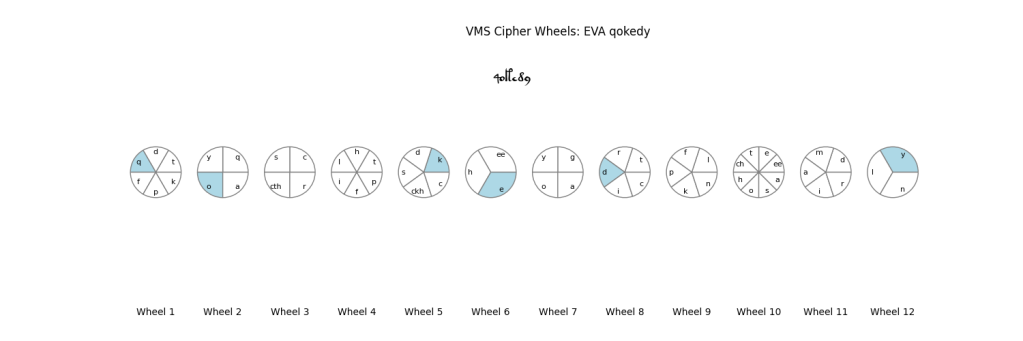

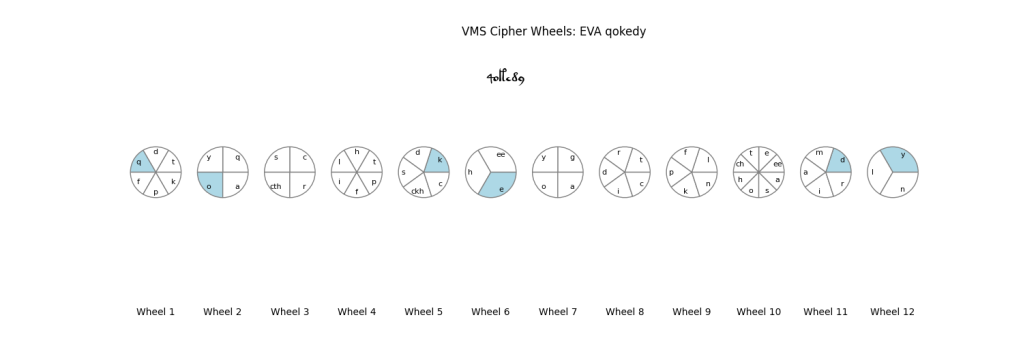
Cipher Wheels – Genetic Algorithm
In my last blog post I talked about a Genetic Algorithm (GA) that works with a set of chromosomes. Each of the chromosomes comprises and is defined by a set of cipher wheels containing VMS glyphs. The goal is to find a chromosome whose set of cipher wheels are able to reproduce as many VMS words as possible, following the rules to be described below.
The hypothesis behind this investigation is that the VMS scribe was using a set of cipher wheels to cipher a plaintext. If the cipher wheels can be identified purely by looking at their output then it would allow further investigations such as what their positions would need to be for each word along the lines of the folio, whether there is a preferred set of wheels for Currier A and Currier B, whether there is a set of wheels that fit the labels better, and so on.
When each chromosome is created it is assigned a number Nwheels of cipher wheels, where 2 < Nwheels < 13. For each of the Nwheels, the chromosome places a number of glphs or glyph groups, Nglyphs, around the wheel, where 2 < Nglyphs < 25. The glyphs are taken at random from the following EVA set:
q d l r s n x i m g c s ee a y o e h ch sh ee in iin t p k f cth cph ckh cfh
Master glyph groups
I’ll refer to the above as “glyph groups”: most are single glyphs but some are groups of two or three glyphs. (The above set is the Stolfi Core/Crust/Mantle EVA glyphs, plus a couple of extras like “in” and “iin”.) The initial population of chromosomes is of size P (typically 200).
Here is an example chromosome showing its 8 wheels of 8 glyph groups (this is just one chromosome from the population):
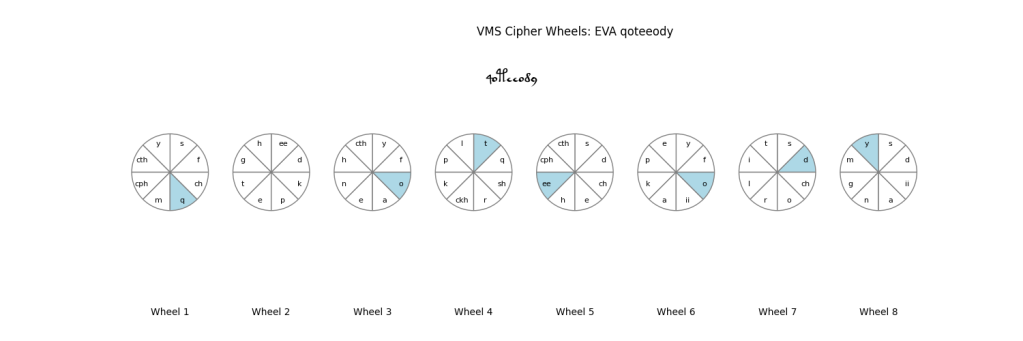
A successful chromosome will be able to encode any word from the VMS, using the following prescription:
- The wheels are used from left to right
- Each wheel may skipped, or it can be used more than once
In the example given above, the VMS word qoteeody is encoding by selecting “q” from Wheel 1, nothing from Wheel 2, then “o”, “t”, “ee”, “o”, “d” and “y” from Wheels 3 to 8.
Here’s a different chromosome:
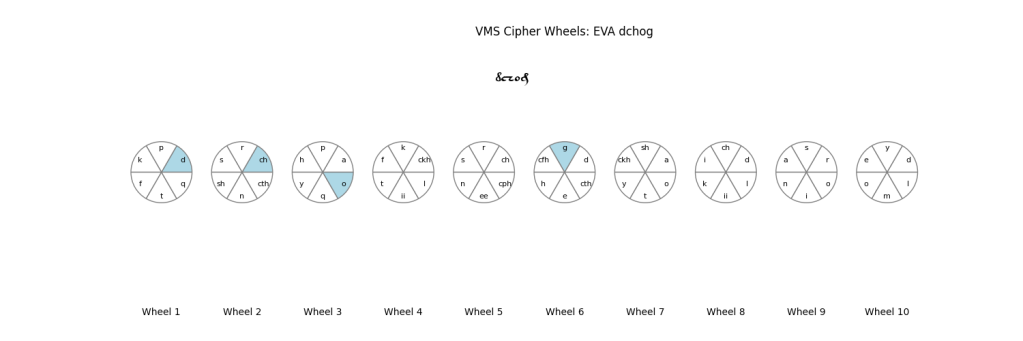
This chromosome has 10 wheels, each of 6 glyph groups. (The chromosomes are not required to have the same number of glyph groups in each wheel.)
How does it work?
Each chromosome is assigned a score, by measuring how well it is able to generate a set of VMS words. The score is affected by the following metrics:
- How many VMS words it can successfully reproduce
- The number of duplicate glyph groups amongst the wheels (fewer is better)
- The number of glyph groups per wheel – a uniform distribution amongst the wheels is preferred
- For each VMS word, the number of glyph groups selected from each wheel – none or one is preferred
Once all chromosomes in the population of size P have been assigned a score, they are ordered by decreasing score. The top half of the population is retained. The remainder are replaced by P/2 new chromosomes, created as follows:
- Crossover: Take two randomly selected chromosomes from the retained population and mate them together. Suppose we select chromosomes C1 and C2: the mating procedure is to select a wheel at random in each of C1 and C2, then select a set of random glyph groups (slices) from each of the selected wheels, and swap them over. So, C1 accepts a donated set of slices of one of C2’s wheels, and C2 accepts a donated set of slices from C1’s wheels. The donated slices are removed from the donor. Chromosomes C1 and C2 are added to the population.
- Mutation: with some probability, select a wheel at random in C1, and a glyph group at random in the wheel, and replace it by one of the master glyph groups. Do the same for C2.
- Add Wheel: with some probability add a cipher wheel to C1, of a randomly selected size, and with a randomly selected set of glyph groups. Do the same for C2.
- Add New: add Nnew (typically 10) freshly generated chromosomes to make the population have size P. This inserts fresh blood into the population.
With the new population, a score is assigned to each of the chromosomes, and the process repeats. In fact there is an intermediate step called “Cull”, which cleans up a bit:
- For each glyph group in each wheel in each chromosome, calculate the number of times that glyph group was used to successfully create a VMS word. If that number is less than a threshold value MinUses (typically 10) remove the glyph group from the wheel. This cleans up the wheels so that do not contain slices/glyph groups that are rarely used.
- For each chromosome, remove any wheel that has no slices/glyph groups left.
The process above defines one Epoch. The GA is allowed to run for many Epochs, but is stopped when the best chromosome (the one with the highest score) doesn’t change over several Epochs.
At the end of each Epoch, the GA prints out some status information, for example:
Epoch 916 Best score 979.994 Worst -310.009 Good words 2699 / 2814 [0, 0, 1, 2, 1, 5, 8, 172, 9, 2, 0, 0]
This shows that at Epoch 916, the chromosome with the best score (979.994) was able to successfully reproduce 2699 of the 2814 VMS words it was presented with. The worst chromosome had a score of -310.009. The list of integers shows the distribution of wheel numbers across the chromosome population: 172 have 8 wheels, for example.
In the next post I will show some results.